We are broadly interested in all the ways in which computational approaches can drive biological discovery.
We develop statistical and machine learning methods and software tools for the analysis of high-throughput sequencing and cellular imaging data, with a focus on single-cell genomic data. We are also interested in the ways in which DNA variation contributes to variation in gene expression at the level of individual cells. We study “single-cell genetics” in this sense by looking at single-cell quantitative trait loci and at the effects of somatic mutations in healthy ageing and cancer.
Recently, we have expanded our research into deep learning methods for the analysis of medical image data. In particular, we work closely with colleagues at St Vincent’s Breast Screen Clinic, the University of Melbourne and the University of Adelaide to identify breast cancer in mammogram images using artificial intelligence with the aim of transforming population breast cancer screening.
Current research projects
-
Analysis methods for single-cell geneticsClonal cell populations at the single-cell levelSingle-cell quantitative trait locus mappingBioinformatics software developmentDeep learning for medical image data
People
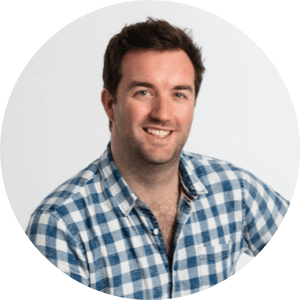
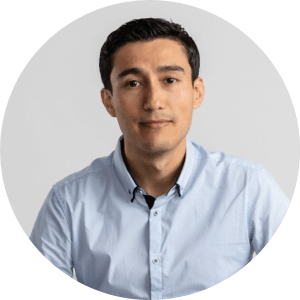
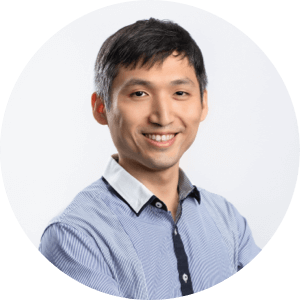
- Michael Elliott, Applied AI Engineer
- Katrina Kunicki, BRAIx Project Manager
- Jarny Choi, Bioinformatician
- Ruqian Lyu, Research Officer
- Shawn Mo, Research Officer
- Millie Dunstone, Research Assistant
- Patrick Gibbs, Research Assistant
- Sagrika Chugh, PhD student
- Neke Ibeh, PhD Student
- Aaron Kwok, PhD student
Lab alumni
- Brendan Hill, Research Assistant
- Sam Tanner, Masters of Bioinformatics Student (now PhD student at The University of Melbourne) https://www.melbournebioinformatics.org.au/people/sam-tanner/
- Tianzhi Yu, Intern (now PhD student at Monash)
- Jeffrey Pullin, Research Assistant (now Statistician at Victorian Department of Health)
- Dr Christina Azodi, Postdoctoral Fellow (now at Bayer Ag)
Selected publications
Frazer, H. M. L.*, Peña-Solorzano, C. A.*, Kwok, C. F.*, Elliott, M.*, Chen, Y., Wang, C., Lippey, J., Hopper, J., Brotchie, P., Carneiro, G., & McCarthy, D. J. (2022). AI integration improves breast cancer screening in a real-world, retrospective cohort study. medRxiv (p. 2022.11.23.22282646). doi.org/10.1101/2022.11.23.22282646
Lyu, R., Tsui, V., Crismani, W., Liu, R., Shim, H., & McCarthy, D. J. (2022). sgcocaller and comapr: personalised haplotype assembly and comparative crossover map analysis using single-gamete sequencing data. Nucleic Acids Research. doi.org/10.1093/nar/gkac764
Azodi, C. B., Zappia, L., Oshlack, A., & McCarthy, D. J. (2021). splatPop: simulating population scale single-cell RNA sequencing data. Genome Biology, 22(1), 341. doi.org/10.1186/s13059-021-02546-1
Cuomo, A. S. E.*, Alvari, G.*, Azodi, C. B.*, single-cell eQTLGen consortium, McCarthy, D. J.^, & Bonder, M. J.^ (2021). Optimizing expression quantitative trait locus mapping workflows for single-cell studies. Genome Biology, 22(1), 188. doi.org/10.1186/s13059-021-02407-x
Lyu, R.*, Tsui, V.*, McCarthy, D. J.^, & Crismani, W.^ (2021). Personalized genome structure via single gamete sequencing. Genome Biology, 22(1), 112. doi.org/10.1186/s13059-021-02327-w
McCarthy, D. J.*, Rostom, R.*, Huang, Y.*, Kunz, D. J., Danecek, P., Bonder, M. J., Hagai, T., Lyu, R., HipSci Consortium, Wang, W., Gaffney, D. J., Simons, B. D., Stegle, O., & Teichmann, S. A. (2020). Cardelino: computational integration of somatic clonal substructure and single-cell transcriptomes. Nature Methods, 17(4), 414–421. doi.org/10.1038/s41592-020-0766-3
ORCID profile: https://orcid.org/0000-0002-2218-6833
Google Scholar profile: https://scholar.google.com/citations?user=A1F5_UEAAAAJ&hl=en
Related news
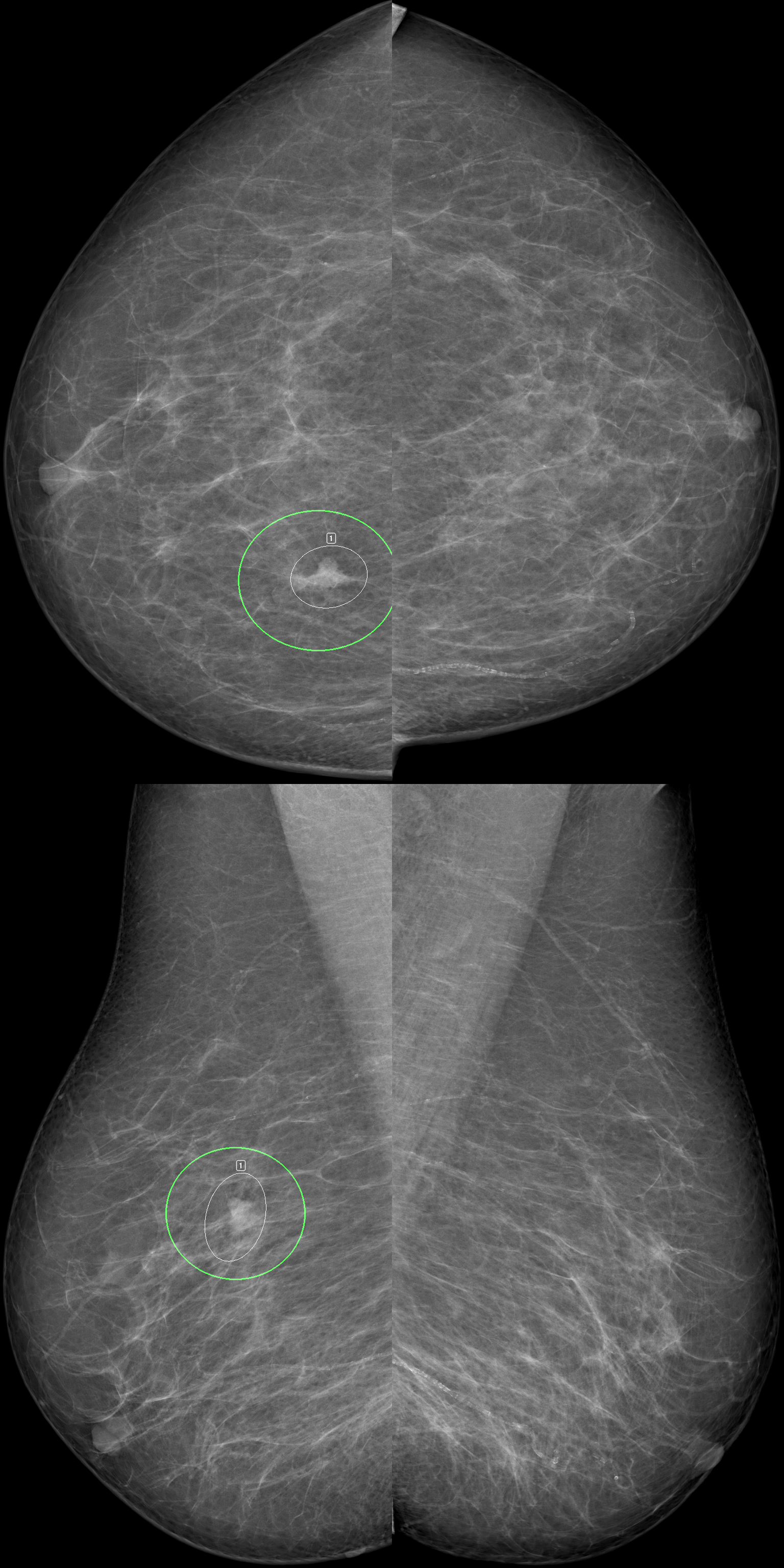
October 2024
AI helps boost breast screening accuracy
Research shows the benefits in integrating AI to read mammograms
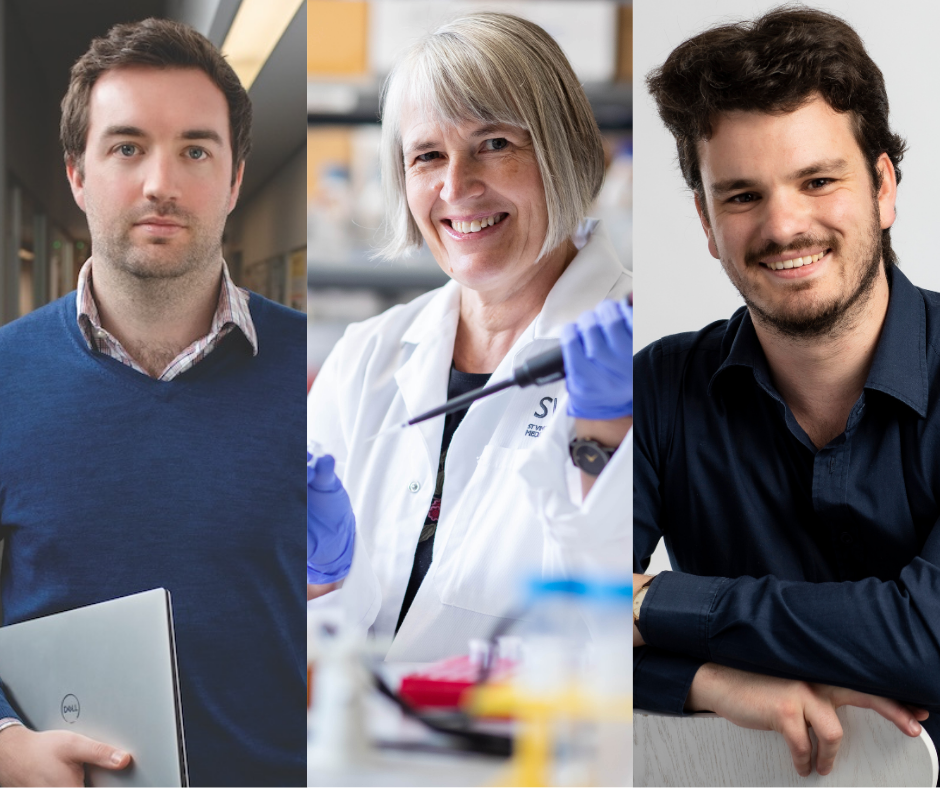
August 2024
SVI’s August Highlights
A wrap up of our latest achievements. Congratulations to Davis, Jarmon, Louise, Vita and Li.
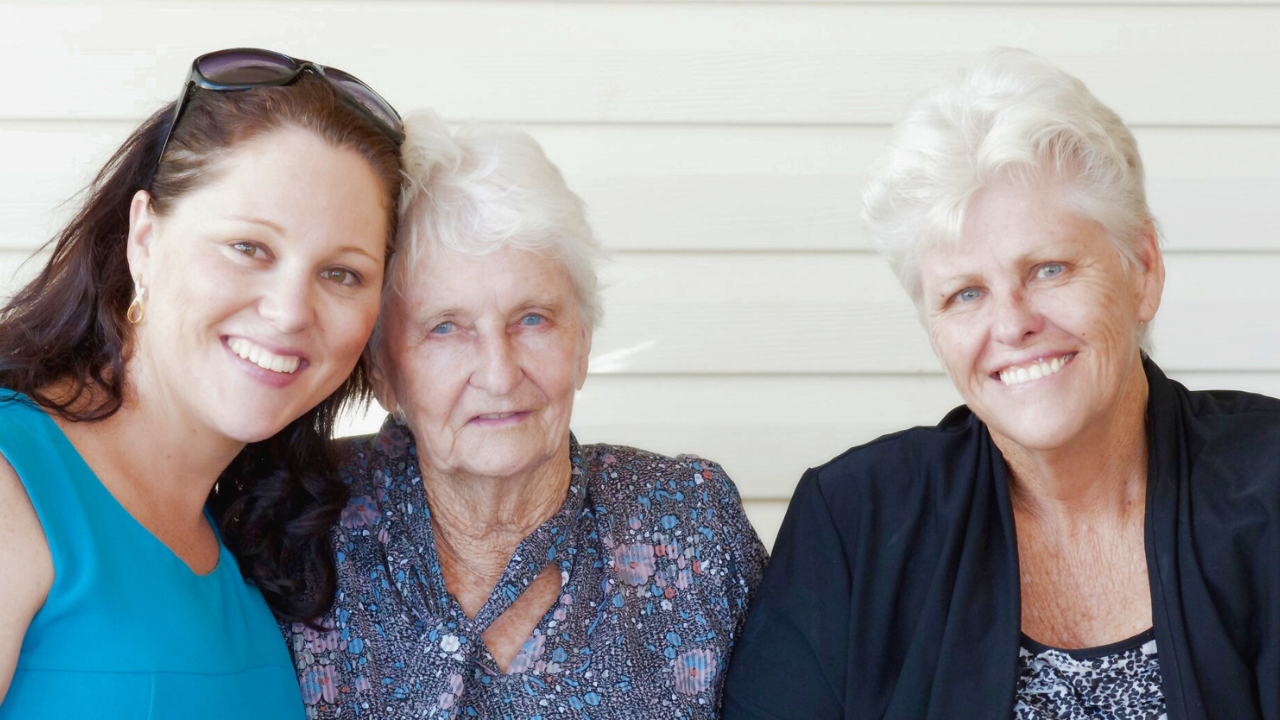
April 2024
Every breath you take
Davis and his collaborators have recently published a study in Nature Genetics, which they hope will help better understand what goes wrong in IPF, with the potential to further the development of therapies that treat the causes of the disease, as opposed to its symptoms.