The research student project aims to revolutionise the study of ovarian biology by employing state-of-the-art machine learning techniques to analyse histological images of ovaries and oogenesis. Through the development of an advanced deep learning model, the student seeks to automate the identification and quantification of various cellular structures and stages of oogenesis within ovarian tissue sections.
This innovative approach will provide a comprehensive and efficient assessment of oocyte development, allowing for a deeper understanding of the molecular and cellular processes underlying normal and perturbed oogenesis. By creating a well-annotated dataset, the student will train the machine learning model to detect specific patterns and abnormalities associated with oocyte maturation, offering valuable insights into female fertility and reproductive health.
This transformative interdisciplinary project has the potential to advance our knowledge of ovarian biology and could lead to the development of targeted interventions to address infertility and reproductive disorders in women.
Supervised by
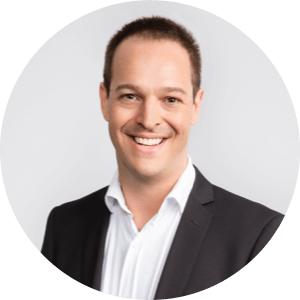
Available for Student Supervision