The research student project aims to harness the capabilities of machine learning to analyze histological images of testes and spermatogenesis. By employing cutting-edge deep learning techniques, the student intends to develop a robust and automated system for accurately identifying and quantifying various cellular structures and stages of spermatogenesis within testicular tissue sections.
This novel approach will enable a comprehensive and efficient evaluation of sperm development, providing insights into the molecular and cellular processes underlying normal and aberrant spermatogenesis. Through the creation of an extensive annotated dataset, the student will train the machine learning model to recognise specific patterns and abnormalities associated with spermatogenic defects, contributing to advancements in male infertility diagnostics and reproductive health research.
This interdisciplinary project holds great potential for unraveling the complexities of spermatogenesis and may lead to the development of targeted interventions to improve male fertility and reproductive outcomes.
Supervised by
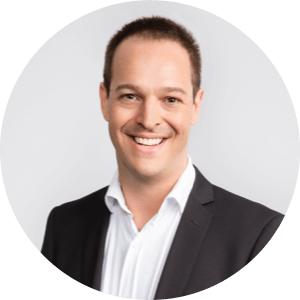
Available for Student Supervision
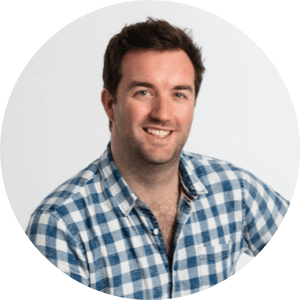